Above-Ground Biomass (AGB) is a crucial forest biophysical property, serving as a key indicator of carbon storage and sequestration in forested ecosystems. It plays a fundamental role in global climate change mitigation, particularly in tropical regions, where forests act as vital carbon sinks.
A recent study (here) by Melitha et al. (2024), published in the International Journal of Earth Science and Informatics, leveraged machine learning and UAV-RGB data to enhance AGB estimation in Miombo woodlands. The research applied four machine learning models; Support Vector Machine with Radial Basis Function (SVM-RBF), Extreme Gradient Boosting (XGBoost), Random Forest (RF), and Gradient Boosting Machine (GBM)—to assess AGB.
The study was conducted across five Miombo woodland sites in the Morogoro Region, Tanzania—four within Village Land Forest Reserves in Kilosa District and one in Kitulang’h’alo Forest, owned by Sokoine University of Agriculture (SUA).
Key Findings
The results demonstrated that Random Forest (RF) was the most effective model, explaining 77% of the variance (R² = 0.77) with an RMSE of 48.7 Mg/ha. XGBoost followed, achieving R² = 0.65 and RMSE = 52.9 Mg/ha. In contrast, GBM and SVM underperformed (R² = 0.28 and 0.29, respectively), likely due to their limitations in handling small, complex datasets.
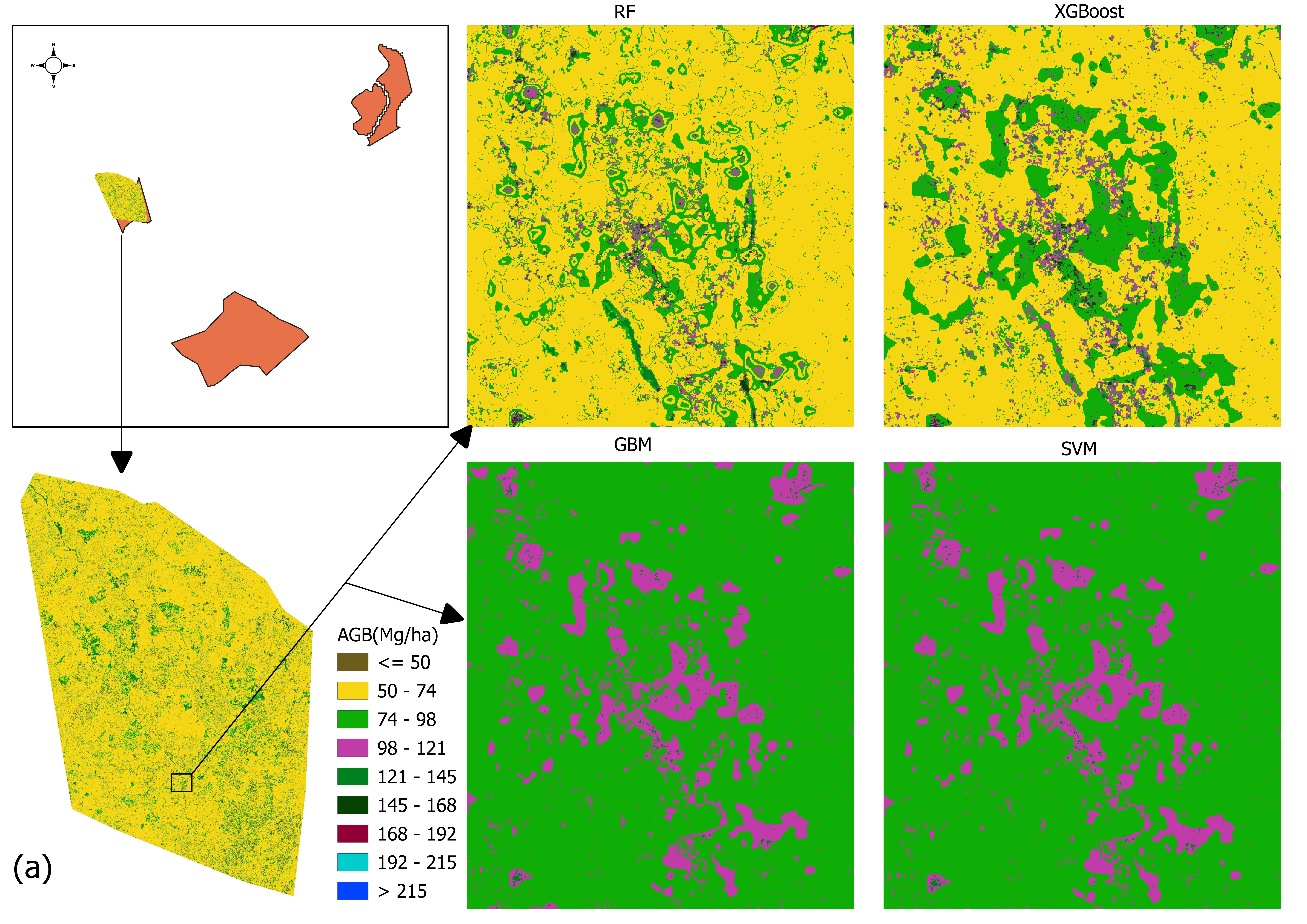
Additionally, the study incorporated AGB mapping, revealing substantial spatial variability across the study sites. The AGB maps indicated that most regions contained biomass levels between 74 and 145 Mg/ha, with hotspot areas exceeding 192 Mg/ha.
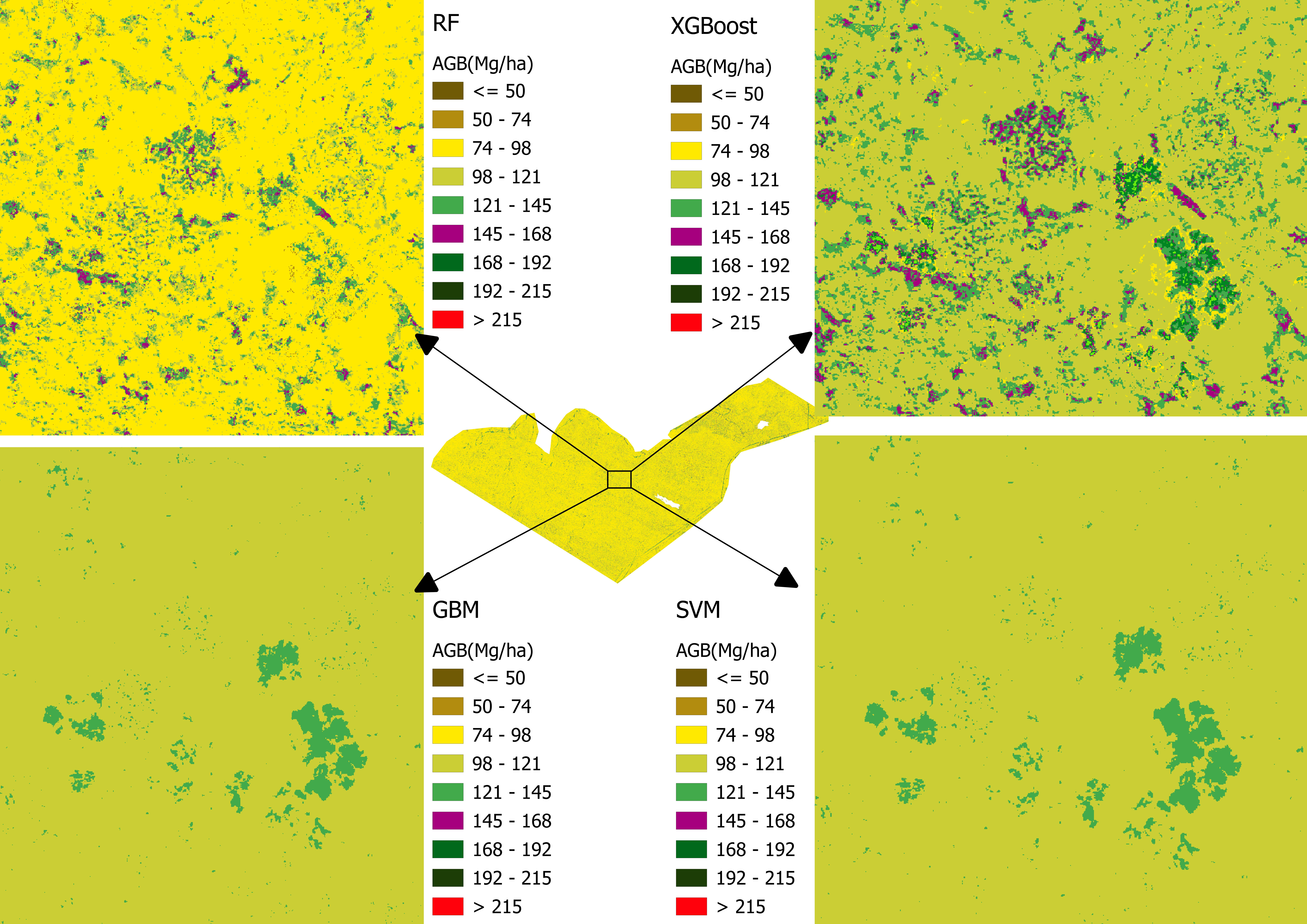
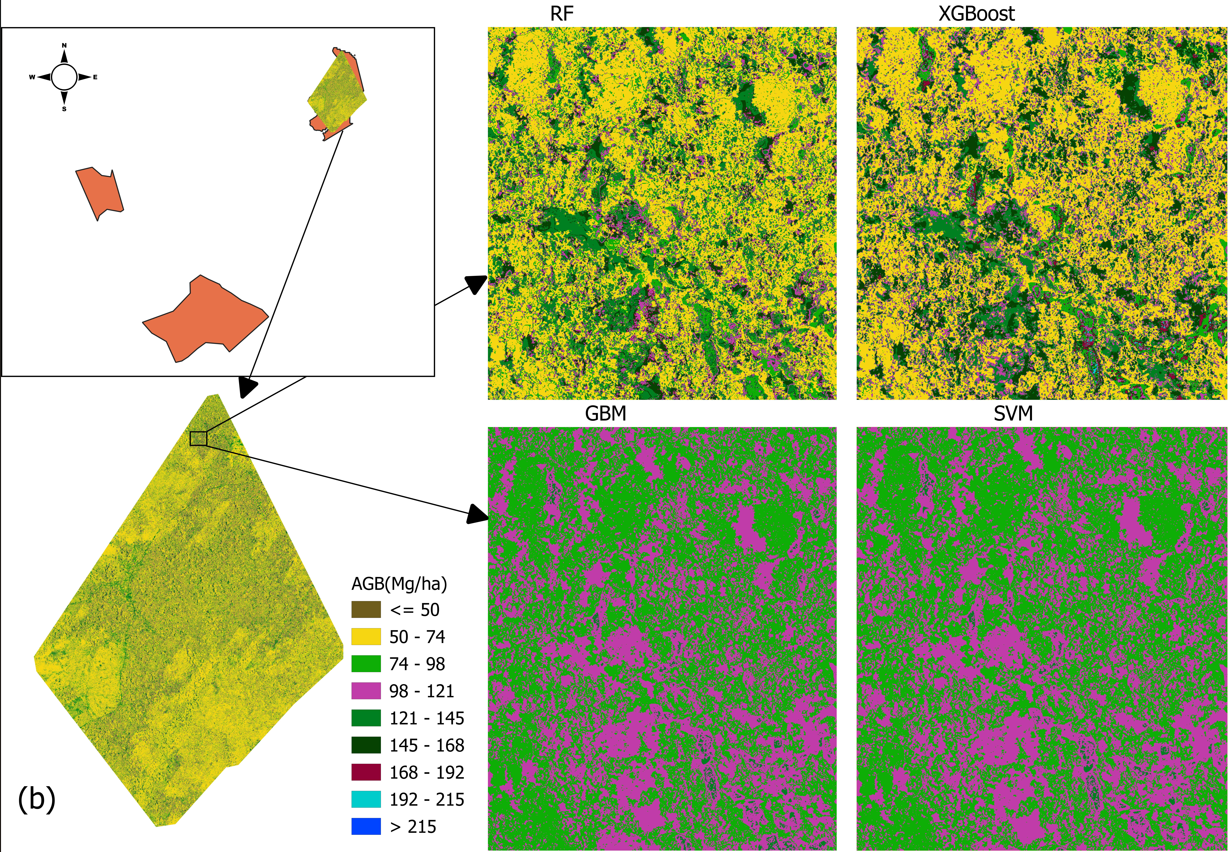
Significance and Future Implications
This study which was funded by Sokoine University of Agriculture (SUA) and supervised by Prof. Japhet Kashaigili and Dr. Wilson Mugasha, represents a pioneering application of machine learning and UAV technology in Tanzanian forestry research. It provides a strong foundation for future studies in remote sensing, artificial intelligence, and precision forestry in Tanzania, offering new pathways to enhance AGB estimation and the assessment of other forest biophysical properties.
Note: Given the efficiency and adaptability of UAV technology, particularly in challenging and remote landscapes, integrating it with modern advancements in machine learning and artificial intelligence (AI) could address various applications in forestry.
As global research in AI-driven environmental monitoring continues to evolve, Tanzania must position itself at the forefront of these cutting-edge technologies to drive innovation in sustainable forest management and climate resilience